Mainly on Personal Genome Industry, Preventable Medicine, Mobile healthcare tech, and Bioinformatics.
Thursday, November 29, 2007
Effect of gene mutation on phenotype
Gene mutation이 phenotype에는 과연 어떤 영향을 미칠까?
이는 SNP 연구의 핵심이 되는 질문이다.
Species간, 각 human 개체간의 genetic variation이
어떤 영향을 끼쳐, species간 혹은 개체 간의 variation을
만들어 낼까?
이 논문에서는 protein complex를 이용해 gene mutation
의 phenotypic effect를 설명한다.
Blast options for ortholog gene finding
서로 다른 두 species 간의 ortholog 를 찾는 방법은
Reciplocal best blast hit,
Reciplocal best distance hit
방법 등 여러가지가 있는데, blast를 이용한 방법이
사용의 편의성에 기인해 널리 쓰인다.
위의 논문에서는 Blast option을 바꾸는 것 만으로
ortholog finding accuracy를 높이는 방법을
제안한다.
Tuesday, November 27, 2007
왜 장기 투자를 해야 하는가?
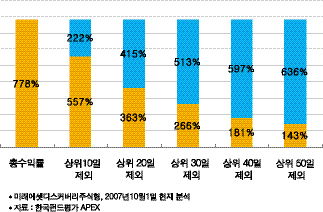
국내 주식 시작에 투자하는 대한민국 대표펀드
미래에셋 디스커버리주식 1호는 현재까지 약 778%의
수익률을 마크하고 있다.
펀드가 시작된 2001년에 1억을 넣어놓고, 그대로 유지하기만
했어도 현재 약 8억 8천만원으로 불릴 수 있었다는 얘기다.
같은 돈으로 같은 기간 동안 수익률이 많이 오를 때 마다 넣었다
뺐다를 반복한 사람의 수익률은 어떨까? 얼핏 생각해서 많이 올랐을 때
팔고, 많이 떨어졌을 때 다시 매수를 한다면 그냥 넣어두고 있었던
것 보다 더 좋은 수익률을 기록할 수 있지 않을까 생각하기 쉽지만,
이는 주가가 언제 오르고, 떨어질지를 정확하게 예측할 때만 가능한
한 마디로 신이 아니고는 할 수 없는 일이다.
이런 식으로 투자를 하면서 주가가 많이 올랐던 상위 10일만을
투자 하지 못했다고 한다면 어떨까? 차트에서 보는 바와 같이
220% 가량의 수익률이 날아간다. 상위 20일, 30일 로 가면
수익률이 절반에도 못 미친다는 것을 알 수 있다.
7년여의 기간 중 단 수십일 펀드 투자를 계속 유지하느냐,
하지 않았느냐에 따라 엄청난 수익률의 차이가 발생하는 것이다.
투자의 기본은 장기 투자, 분산 투자다.
문제는 이 기본을 지킬 수 있는가다.
매일매일의 신문 기사와 증시 시황에 흔들리고
불안에 떨 만큼 '투자'가 불안하다면
안전한 은행에 넣어놓고 콩고물 같은
이자만 받으며 만족해야 할 수밖에 없다.
Wednesday, November 21, 2007
Fun&Co
두 그룹의 microarray data set의 functional difference를
비교하고자 할 때 ( e.g. 서로 다른 tissue, disease vs. normal 등)
사용할 수 있는 방법으로 fun&co 이라는 web server가 공개되었다.
Procedure는 아래와 같다.
1. 각 그룹의 intensity를 rank로 변환
2. 가능한 모든pair probe set에 대해 spearman correlation coefficient 계산
3. pair probe set이 동일한 gene을 detection하는 경우가 아닐 때, 연관된 GOmain term을 counting
4. 각 그룹의 counting 된 GO term set을 비교하여, 그룹과 Over-correlated or under-correlated 된 GO 를 선정
다른 방법들과 구분되는 특이한 점이라면,
하나의 GO term을 지칭하는 Pair gene이
correlation되는 경우 연관된 GO term에만
의미를 두었다는 것이다.
이는 multi data set comparision의 경우 몇가지 advantage를 가지는데
1. 각 data set에서 DEG test를 할 필요가 없다는 점
2. 따라서 normal condition에 대한 고려가 필요없는 경우 ( 서로 다른 tissue 비교와 같은 경우), 각 data set에서 관련된 array만 고려하면 된다는 점
등이 된다.
Tuesday, November 20, 2007
Human PAML Brower
이용해 계산한 결과를 담은 DB가 공개 되었다.
Human PAML Browser : http://mendel.gene.cwru.edu/adamslab/pbrowser.py
Human gene은 약 22,000~28,000 로 추정되는데,
여기서는 UCSC multispecies genome alignment에
의해 얻어진 ortholog 13,721개에 대한 결과를 담고있다.
PAML을 돌리기 위해 DNA sequence pair, Protein sequence pair
들을 각각 준비해야 하고, computation time도 꾀나 걸린다는
점을 생각해 볼 때, 필요할 때 마다 관심있는 gene의 PAML
결과를 검색할 때 유용할 것 같다.
NAR paper : http://nar.oxfordjournals.org/cgi/reprint/gkm764v1?ck=nck
Monday, November 19, 2007
CellMontage : Gene expression alignment tool
Gene expression 정보가 Public database인 GEO와 ArrayExpress에
축적되는 양이 점점 많아지면서, DB에 들어있는 expression 정보에의
접근이 중요한 Research topic으로 떠오르고 있다.
Sequence alignment와 마찬가지로, 특정한 Gene expression profile과
비슷한 Profile들을 찾고, 묶어내고 하는 일련의 과정들이 바로 이 topic의
목적이 된다.
Bioinfo2007에 발표한 내 논문에서는 실험 단위의 Gene expression 정보들을
clustering하는 방법을 논의했는데, 여기서는 이 보다 작은 sample 단위의
expression profile을 clustering하는 방법이 고안되어 있다.
하나의 실험 하에서 수행된 모든 expression profile들은 모두 그 실험의
고유한 목표 아래, 고유한 실험 환경에 따른 expression의 uniqueness를 가지고 있고,
따라서 gene expression profile의 유사성을 찾기 위해서는 각 sample들 간의
similarity 보다는 실험 단위의 level에서의 유사성을 찾는 것이 더 의미있지
않을까 했던 것이 내 생각이었다.
이것이 더 의미있다는 것을 보일 수 있다면 또 하나의 좋은 논문 거리가 될 것 같다.
Sunday, November 18, 2007
NetworkAnalyzer
Network의 다양한 physical topology 들을
한꺼번에 계산해주는 NetworkAnalyzer가 공개되었다.
NetworkAnalyzer 홈페이지: http://med.bioinf.mpi-inf.mpg.de/netanalyzer/index.php
Cytoscape 상에서 실행시키면 아래와 같은 계산 결과 창이 뜬다. 각 Graphic은
다양한 size로 export가 가능하다.

Monday, November 12, 2007
BioLinux course
University of cofenhagen에서 개설된 course로
Bioinformatics 연구 시, OS로 널리 쓰이는 Linux
의 이용과 다양한 bioinformatics approache 이용을
목표로 함.
Thursday, August 9, 2007
R fuction : sort와 order
이들을 특정 값을 기준으로 데이터를 정렬해야할 필요가 있다.
예를 들어,
>pvalue=c(0.1,0.001,0.003,0.0023,0.3)
와 같은 pvalue를 저장한 list가 있다고 하면 sort와 order 함수는 아래와 같은 결과를 돌려준다.
> sort(pvalue)
[1] 0.0010 0.0023 0.0030 0.1000 0.3000
> order(pvalue)
[1] 2 4 3 1 5
즉, sort는 값 자체를 sort한 결과를, order는 값을 기준으로 sort한 결과를 list의 atom 순서로 돌려준다.
The secret
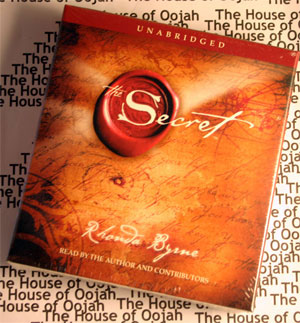
최근 미국에서 베스트셀러 5위 안에 꾸준히 랭크되고 있는 책, The secret 을 읽었다.
원서가격 14,000이면 조잡한 페이퍼백의 수준을 겨우 넘긴 간지 안 나는 모양새의 책들이
대부분인데 반해, 화려한 코팅지(?)와 올컬러의 동급 최고의 미모를 지녔다.
책은 The secret을 설명한다. 뭔고하니, 행복하고 성공적으로 자신이 원하는 삶을 도대체
어떻게 하면 살 수 있는가에 대한 '비밀'. 사실 이런 주제의 책은 널리고 널렸다. 또 대부분의
그런 부류의 책들의 내용도 거기서 거기다.
'너 자신을 믿어라'
'긍정적인 생각을 해라'
'원하라, 그럼 이루어 질 것이니...'
등등의 책을 읽는 순간엔 힘을 주지만, 또 이미 알고 있는 사실이지만, 실천이 어려운
그런 하나마나인 말들로 가득한, 읽고 나면 왠지 속았다는 느낌이 드는 그런 내용들로
일관하는 종류의 책이다.
그런데 왜 갑자기 이 책이 베스트셀러가 됐을까? 글쎄 여러가지 이유가 있을 것 같은데,
한 가지는 이 책이 먼저 TV 다큐멘터리로 제작되어 큰 반향을 일으켰다는 사실이 아닐까?
미국에선 영화 흥행만 하면 그 영화에 관련된 소설 등도 덩달아 베스트셀러의 반열에 오르곤
하는데, 아마 그런 문화적 기반 속에서 책이 잘 나가고 있지 않나 생각된다.
어쨌거나, 이 책의 내용은 현재의 나에겐 시종일관 쓸데없는 잡 생각, 부정적인 생각은
버리라고 충고한다. 항상 재미있는 일이 아니면 의미없는 일이고 할 필요도 없다고
하고 싶고 재미있는 일을 해야한다고 부르짓고 다니지만, 실상은 지금까지 살아온
매너리즘에 빠져 그렇고 그런 하루하루를 살아가고 있는 나 아니던가?
If you want, you deserve it.
If you don't want, you still deserve it.
원하는 걸 갈망하면 이뤄진다.
원하지 않는 걸 갈망해도 역시 이뤄진다.
'이런 삶을 살기 싫어'
'이런 여자는 싫어'
'그 놈은 정말 짜증나'
'돈 없는 거지같은 삶은 싫어'
이렇게 부정적인 갈망을 해도, 그것은 희망이 이루어지는 것과 마찬가지의 매카니즘으로
현실이 된다는 얘기.
The law of attraction. 이것이 The secret의 내용을 한마디로 압축할 수 있는 문장이다.
Thursday, July 26, 2007
R에서 heatmap
microarray data를 읽어온 파일의 경우 '., (, ) ' 등의 특수 문자가 label name 즉 row or column name이 되는 경우에 R에서는 이를 그대로 '.'으로 치환하는데, 이렇게 치환된 name은
heatmap을 그리면 표시가 되지 않을 때가 있다. (정확한 원인은 아직 모른다, 될 때도 있고 안 될 때도 있으니... 이건 뭐...)
label에 표시되지 않는 name의 예 : Control(D)_2
아래 그림 : column에 label이 표기가 빠져있는 heatmap
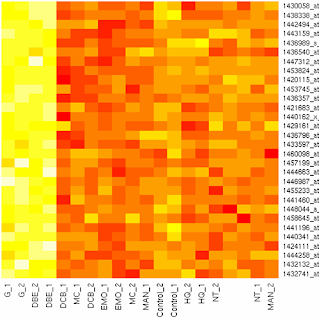
Wednesday, July 11, 2007
libaffy : Affymetrix processing programe
User interface로 손쉽게 가장 널리 사용되는 affymetrix processing 방법 MAS와 RMA를 이용할 수 있는 프로그램. CEL 파일과 CDF 파일을 인풋으로, 아웃풋은 processing된 파일. DEG selection 과 같은 추후 작업 기능은 제공되지 않는다.
R+Bioconductor 에 비해 장점은 메모리를 덜 먹고 약간 빠르다는 것. 수십장의 array의 경우 메모리 문제로 processing이 안 되는 경우가 있는데, 이를 해결해 준다고 논문에 지껄여져 있다.
Tuesday, July 10, 2007
Top journal의 article은 뭐가 특별한가?
Protein interaction network의 hub protein들은 interaction type에 따라 party hub와 date hub로 나뉘는데, 이 때 party hub는 protein complex 처럼 함께 interaction하며 존재하고 기능하는 protein들이고, date hub는 cell signalling의 hub protein으로 기능하여 여러가지 protein들과 시간과 장소에 따라 interaction하는 protein이다.
여기에 더해 이전까지 리포팅 된 내용은 party hub와 date hub의 evolution rate의 차이, protein network과 관계된 내용은 아니지만, protein structure의 특성과 evolution rate의 관계 정도 였다.
내 석사 졸업 논문도 바로 이 protein structure 특성과 evolution rate의 관계에 관한 further study였고, 이 점이 또한 이 science 논문에 관심을 증폭시킨 원인이기도 하다.
논문을 읽으면서 난 당연하지... 당연하지... 를 연발했는데, 이 topic에 관심있는 연구자라면 누구나 그런 반응을 기대할 수 있을 만큼 당연한 연구에 당연한 결론이었기 때문이다. 그런데 어떻게 이 논문이 science에 게재될 수 있었을까?
나름대로 의견을 제시해 보자면,
첫째, Gerstein 이라는 protein network 연구의 대가 랩에서 행해진 연구라는 것
둘째, 누구나 알고 있는 and 이미 밝혀진 두 가지 topic을 통합하여 새로운 insight를 제공할 수 있는 연구라는 것 정도가 될 것 같다.
두번째 이유보다는 당연히 첫번째 이유가 Big journal publish에 중요함은 다시 말할 필요가 없을거다. 만약 내가 같은 논문을 써서 science에 submit 했다면 당연히 reject되었을 거고, 논문의 quality로 보자면 Bioinformatics, Plos computational biology, NAS 회원의 도움이 있다면 PNAS 정도가 maximum이지 않았을까...
개인적으로 top journal에의 출판을 별로 중요하게 생각하진 않지만, publication list가 나중의 사회적, 경제적 위치의 결정에 관여하는 한국 사회에 살고 있다보니 이런 문제에 관심이 가는건 어쩔 수 없다.
Monday, July 2, 2007
Wanted Video clips in google
authors@google을 검색하면 Bestseller나 이슈가 되는 책의 저자들의 구글에서의 강연 video들이 검색되어 나온다.
techtalk 은 분야를 막론한 다양한 science/technology의 연구자들의 구글 세미나가 검색된다.
Sunday, June 24, 2007
Flow: The Psychology of Optimal Experience
My answer to the question is that only when we do what we love to do and enjoy. Success is also the result of this way of life. Anyone who do what they enjoy can be happy. But not all of them achieve success in terms of material world. People just think that people with bunch of visuable things did success. When they say so, they only concern about the success in society. But we can't say that a person is successful if s/he think they are not happy.
My definition of success is the same as being happy. How to be happy and successful at the same time then? The book 'Flow: The Psychology of Optimal Experience' answers to this question.
Tuesday, June 19, 2007
Perl programming common error
Exam)
@data=('Good_haha','Bad_haha');
for $i(@data)
{
$i=~s/\_haha//g;
}
에서 data array의 변수를 받은 iterater $i에 가해진 정규식 substitution ( _haha 를 잘라낸다)
은 @data의 실제 변수들에 변화를 가한다.
$i는 @data의 변수 그 자체이지, 이를 call by value로 받은 지역 변수가 아니다.
Monday, June 18, 2007
Bioconductor package에서의 DEG finding method 사용시 유의 사항
Package DEDS의 경우
Fold change=mean(Ms)-mean(Mc)
의 공식으로 계산된다. 여기서는 각 Microarray intensity가 이미 log계산을 거친 값이라고 가정하고 있으므로, log(Ms/Mc)의 fold change값을 얻게 되지만, normalization method에 따라 log 계산을 거치지 않은 경우도 많으므로 data type의 check와 각 계산법의 가정의 체크가 필요하다
Sunday, June 17, 2007
MindSet
The core of it is that a person who suceed believe a distinguished ability could be achived by continuous effort while a person who fail believe it's determined when we born.
Yes, that's true. As lots of authors of biography insist that effort can change our life, we can really change our life if we have a passion and effort with a belief that our ability would be improved someday.
Tuesday, May 29, 2007
DEG finding in R
Gene A 100 120 300 500
와 같이 A에 대해 normal sample(검은색)과 control sample(붉은색)의 expression data가 있고, 이를 바탕으로 A gene이 control 환경에 specific 하게 up or down regulation되었는지를 t-test를 통해 알아보고자 한다면, Two sample t-test를 쓴다.
> a=c(100,120,300,500)
>t.test(a[1:2],a[3:4])
M x N의 microarray data matrix에서 column 1:4가 normal 5:8이 control이고 M개의 gene의 t-test를 계산한다면,
>for(i in 1:nrow(matrix))
>{
>value<-t.test(matrix[i,1:4],matrix[i,5:8])
>result[i]=value$p.value
>}
각 gene의 t-test 결과 p-value가 result에 저장된다.
Sunday, May 27, 2007
R for microarray data handling
1. Matrix of F rows and S columns
- F is the no. of features
- S is the no. of samples
2. Feature information matrix
- F X F matrix, each links to standard feature(gene) id in public DB
3. Data table of information on samples ( S X V matrix)
- v is the no. of covariates
와 같이 총 3개의 data representation matrix가 하나의 microarray data를 표현한다.
Reading array data from flatfile
Microarray data를 읽기 위한 R package들이 존재하나, 특정한 data form을 요구한다. 서로 다른 lab or platform or pubic DB 에서 얻어진 data들을 일괄적으로 처리하기 힘들다. 따라서 기본적인 R 기능을 이용한 data reading으로 data을 matrix형태로 읽어들이고, 이를 processing한다.
1. reading expression file
> ex<-read.table('~/file',header .., sep .. )
읽어들인 data를 numeric only data로 변경하고, 이를 matrix 형 data로 변환
>ex_mat=as.matrix(ex)
matrix형으로 변환된 data는 R package 함수에 기본형으로 쓰인다.
Monday, May 14, 2007
Handling Public Microarray Data
Gene은 NCBI, Protein은 SWISS-PROT 처럼 Microarray Experiment도 public domain에 deposit하고 standard format에 맞추어 deposit하고 정형화된 방법으로 쉽게 접근하여 analysis 할 수 있게 하자는 취지로 만들어진 것이 바로 공용 Microarray DB인 NCBI의 GEO와 EBI의 ArrayExpress다.
그러나 Gene과 Protein와 다르게 microarray는 독립된 하나의 biology experiment이기에 실험 결과에 영향을 주는 parameter들이 굉장히 많고 이들 각각을 변인 제어하여 비교하기란 얼핏 불가능해 보이기까지 한다. 실제로 같은 cancer cell의 RNA extract를 가지고 같은 microarray platform에서 실험을 하여도 실험실 마다, 진행한 실험자에 따라 variation이 생기고, 이 variation은 microarray 실험의 목적인 DEG(Differentially Expressed Gene) 구분에도 확연히 다른 결과를 줄 수 있기에, 한 쪽에서는 microarray 무용론까지 제기되는 실정이기도 하다.
So what ?
그럼에도 불구하고 microarray 실험이 제공하는 특정 환경에 따른 expression 정보는 생물학의 패러다임을 변화시키는 한 축을 담당하고 있고, drug discovery, toxic, environmental chemical assessment 등 생물학의 산업화와도 밀접한 관계가 있어, microarray 연구는 더욱 가속화 되고 있는 상황이다.
특히, public microarray DB들의 기하급수적 성장으로, 정교하고 믿을만한 microarray 정보 분석 구축 시스템이 시급한 상황이다.
Bottleneck
Public microarray data의 사용에 가장 큰 문제는 앞서 언급했던 microarray data의 variation에 있다. 특히 같은 환경에서 얻어진 data라도 lab, platform에 따라 결과가 달라 이들 cross laboratory, cross platform data들을 비교 분석할 수 있는 신뢰도 있는 reference DB나 분석 방법의 개발이 필요하고, 이러한 기반이 닦여지고 나면, cross species data 분석, cross condition 분석 등을 통해, 원래 microarray가 목적했던 특정 질병 특정 환경 하에 발현되는 DEG들을 구분하고 이를 바탕으로 Prognostic method개발과 computational toxic compound prediction, understanding genetic behaviors resulting phenotype 등이 가능해 질 수 있을 것이다.
What has been done ?
Cross (platformspecies) microarray data analysis tool
*Integrative Array Analyzer (http://zhoulab.usc.edu/iArrayAnalyzer.htm)
Published Researches
*Leming Shi et al, BMC bioinformatics, 2005, 6,s12
- Cross-platform analysis 를 위해 data의 intra-platform consistency를 측정하기 위해서는 Log ratio가 log intensity에 우선이 되어야 한다. Consistent intra-platform data 선정 후, cross-platform analysis를 해야 consistency가 높아진다.
- 서로 다른 platform의 data를 비교하는 것인 만큼 각 platform에 dependent한 data filtering이 선행되어야 한다. 이 과정이 cross platform validation 가능한 정도의 신뢰도를 가지는 gene set을 결정하게 된다.
- Cross concordance 측정을 위한 gene selection 방법은 p-value 보다 fold change, SAM이 더 정확하며, selection되는 gene 숫자가 적을 수록 효과는 significant.
*Phillip Stafford et al, NAR, 2007, dio:10.1093
- Affy 와 Agil chip을 Liver, Lung, Spleen 세 가지 normal tissue extract로 실험하여 다양한 normalization 방법들을 적용하여 GO 분석과 feature selection 수행, 가이드라인 제시
*Lei Guo, .. , Leming Shi, Nature Biotech, 24,1162
- MAQC project의 toxicogenomics data에 대한 cross-platform analysis
- 앞선 논문에서와 마찬가지로 fold change가 consistent DEG 선정에 가장 좋은 결과를 내었는데, 특정 fold change range ( e. g >2.0, 1.3) 보다 ranking을 이용하는 편이 더 좋은 결과. 이 때, strict하지 않은 P-value cut-off(0.05)로 먼저 전체 gene set을 거른 후, 나머지 gene들에 대해 fold change의 ranking을 선정 특정 ranking 이상의 gene들을 선정하는 방법을 이용하였다.
- GO, KEGG 분석을 통해 biologically meaningful한 결과를 얻을 수 있었고, 결과적으로 cross-platform for toxicogenomics 연구를 뒷받침
Wednesday, April 18, 2007
Role of CNEs
보존된 서열들의 대부분을 차지하지만, 그 기능에 대해서는
거의 알려진 것이 없었다. 실험을 통해 알려진 바로는
gene regulation에 관여한다는 것이 알려져 있지만,
어떤 mechanism을 통해 어떻게 이런 기능을 하는지에
대해서는 역시 아직까지 밝혀진 바가 없었다.
MIT의 Broad Institute의 연구진들은 이들 CNE의
역할을 규명하려는 일련의 실험을 진행하였다.
( http://www.pnas.org/cgi/reprint/0701811104v1)
먼저 CNE 중 비교적 긴 motif (12-22nt)를 찾아내었는데,
이렇게 얻어진 CNE motif는 총 233개, 전체 CNE의 개수는
human genome에서 60,000개에 달했다고 한다.
이어 연구진은 이들 motif의 역할을 실험적으로 규명했는데,
RFX1 protein family가 가장 conservation이 강한 CNE motif와
결합한다는 사실, gene activation을 제한하는 insulator 기능을 가진
CTCF protein의 결합 부위를 결정한다는 사실을 밝혀내었다.
또한 CTCF protein 결합 부위에 의해 분리된 인접 유전자들은
gene expression이 감소되는 경향이 있음을 확인했다.
CTCF protein에 의해 분리된 유전자의 단위들이 gene expression의
발현 정도를 조절하는 잣대가 된다면, 이를 통한 상대적 발현 정도의
차이를 예측하는 시스템의 구축이 가능할 것이고, gene expression
도 protein domain 처럼 묶어 각각의 기능적 특성들을 이해하고 분류하는
일련의 과정들도 가능해 질 것이라 본다.
Microarray 를 통한 gene expression 연구 또한 새로운 insight를
가질 수 있을 것 같다. 무조건 DEG들이 중요한 것들이라기 보다는
어떤 gene context에 속해 어느 정도의 expression을 가질 것인가
라는 맥락 속에서 얼마나 다른 발현 정도를 가져왔느냐가 핵심이
되어야 할 것이다.
Tuesday, April 10, 2007
Interacting with web pages with perl
LWP::UserAgent
주어진 url의 page를 통째로 읽어오는데 쓸 수 있다.
HTML::ContentExtractor
주어진 url의 page에는 메뉴바나 광고등의 사용자가 원하지 않는 정보가 포함되어 있을 수 있는데, 이 때 이 모듈을 이용하면 DOM(Document Object Model) tree분석을 통해 main text의 content만을 extraction할 수 있다.
Tuesday, April 3, 2007
Frequently used commands in R
>install.packages('package name')
For bioconductor package
>source('http://www.bioconductor.org/biocLite.R')
>biocLite('package name')
Reading data
read.table 옵션
row.names=1이면 첫번째 column의 row값이 row name이 된다.
Glimpse of data
>str(data)
>summary(data)
>print(data)
Divide plotting space
>par(mfrow=c(n,m))
nXm 행렬 형태로 plotting 공간을 나눈다.
Distribution model
[rpq][normpoisbinomunif]
각 distribution 에 따라 random generator, cumulative robability, deviate fo currespond cumulative probability.
Length of column and raw of matrix
>nrow(matrix) ; matrix의 row 개수 반환
>ncol(matrix) ; marix의 column 개수 반환
>dim(matrix) ; matrix의 row와 column 반환
>length(matrix) ; matrix의 전체 길이 반환 ( matrix 형 data 인 경우, is.matrix()로 판정 or class())
Boxplot
header가 없는 matrix의 boxplot은 모든 데이터를 하나의 column으로 간주하는데, 이 때 각 column에 대한 boxplot을 그리려면
>boxplot(matrix~col(matrix))
Tuesday, March 20, 2007
Basics of Microarray analysis
A sequence of microarray experiment
- cDNA Spotting
- RNA preparation
- Hybridization
- Scanning
- Statistics
Problems in microarray data analysis
RNA extracts might be different according to which environment and when they were extracted. It's related to false positive signals (DEG).
Platform difference : different set of DEG could be determined between cDNA microarray chip which measure expression pattern from two samples and oligonucleotide chip which measures from single sample (absolute expression level).
Monday, March 19, 2007
Pros and cons of Toxicogenomics
- Prioritization of chemical lists
- Deciphering mechanisms of action
- Identifying biomarkers of exposure
- Identifying biomarkers of toxicity
- Cross-species extrapolations
- Identifying species sensitivities
- Analysis of mixtures toxicity
Toxicogenomics based toxic mechanism prediction
- Used to rank and prioritize the potential toxicity of NCEs in early stage of development
- Toxicities observed in models(mouse, rodent, dog, etc) are not relevant to humans since mechainisms of action are not conserved across species.
Impediment of toxicogenomics
- Lack of uniform study design
- Multiplicity of normalization and analysis strategies
- Questionable reproducibility of microarray data across the platforms
- Semiquantitative nature of proteomics
- Limited availability of metabolite annotation
- Absence of data quality control measures and standards
- Lack of effective data sharing and reporting standards
Toxicogenomics Standards
- www.mged.org
- psidev.sourceforge.net/
- www.smrsgroup.org
Toxicogenomics DBs
Lack the ability to integrate toxigogenomic data across chemical and biological space to mechanical pathways and networks
Sunday, March 18, 2007
Perl IDE programs
http://www.freedownloadscenter.com/Best/perl-gui-ide.html
Thursday, March 15, 2007
Scene 그래프 갱신
오직 BranchGroup만이 프로그램 실행 중에 detach, add 가 가능하기 때문에, BranchGroup의 생성시 이를 지정해 주어야 한다.
Exam code
- BranchGroup branch=new BranchGroup();
- branch.setCapability BranchGroup.ALLOW_DETACH);
- branch.setCapability(BranchGroup.ALLOW_CHILDREN_WRITE);
- branch.setCapability(BranchGroup.ALLOW_CHILDREN_EXTEND);
2번: branchgroup 자신이 parent group에서 분리될 수 있게 선언
3,4번: child group이 확장 되거나 새롭게 add될 수 있게 선언
상호 작용
Parent group과 child group의 branchgroup의 선언이 서로 match가 되어야
제대로 기능할 수 있다.
Exam code
BranchGroup parent=new BranchGroup();
parent.setcapability(BranchGroup.allow_child_write);
...
BranchGroup child=new BranchGroup();
child.setcapability(BranchGroup.allow_detach);
...
parent.addchild(child);
parent.removeall();
앞의 부모 branch에서 자식 branch를 다시 쓸 수 있다고 선언했지만,
아래의 자식 branch에서 스스로 detach 할 수 있다고 선언하지 않으면
parent.removeall()은 동작하지 않는다.
Java3d와 스윙 for layout, 문제와 해결
문제 코드
JFrame frame=new JFrame();JPanel panel=new JPanel();panel.add(BorderLayout.center,canvas);frame.getcontentPane().add(BorderLayout.center,panel);
해결 코드
panel.setLayout(new BorderLayout());
panel.add("Center",canvas);
로 BorderLayout 객체를 생성하여 layout설정 후, 문제가 해결되었다.
Wednesday, March 14, 2007
Simplest java3d program
import ...
public class Basic extends Applets{
public Test2{
SimpleUniverse u=new SimpleUniverse(); // SimpleUniverse 인스턴스
BranchGroup Root=new BranchGroup(); // Root branch group
// Sphere를 만들고, 색 선정 후, BranchGroup 에 추가한다.
Appearance ap=new Appearance();
Color3f=new Color3f(1.0f,0.0f,0.0f);
ColoringAttributes at=new ColoringAttributes(color,ColoringAttributes.shape_flat);
ap.setColoringAttributes(at);
Sphere sp=new Sphere(0.1f,ap);
// 만들어진 sphere를 Branchgroup Root에 연결
Root.addChild(sp);
// Root를 simpleuniverse에 연결
u.addBranchGraph(Root);
// Viewer조절
u.getViewingPlatfrom().setNominalViewingTransform();
}
public static void main(String[] args){
//Applet 스타트
Basic b=new Basic();
}
}
색 지정의 우선순위
- Shape3D나 도형 primitive class(Box, Cone, Sphere, etc) 들은 Appearance 와 Material 클래스가 외형을 정의한다.
- 색 지정의 경우, 두 클래스 모두에서 가능하나, Material 클래스가 우선이다. 따라서 Material class에서 이미 색 지정이 되어 있다면, Appearance 클래서의 색 지정은 무시된다.
Java3d API for Material class
Exam
- Appearance ap=new Appearance();
- Color3f red , black, white, green ...
- Material m=new Material(red,black,white,green,80.0f);
- ap.setMaterial(m);
Wednesday, March 7, 2007
Common errors in Java programming
문자열을 읽어 형변환을 하는 경우
str.substring(23,26)을 읽어 정수형으로 형 변환.
int a=Integer.parseInt(str.substring(23,26));
이 때, 첫 문자가 공백문자인 경우, 에러 발생.
str.substring(23,26).trim() 을 써서 공백문자를 제거한다.
Tuesday, March 6, 2007
Java3D - Geometry
1. By geometric utility classes
2. By specifying the vertex coordinates
3. By geometry loader
Monday, March 5, 2007
Java3D-Very basics
1. Download Java3D distribution provided by http://java.sun.com/products/java-media/3D/download.html
2. Install Java3D with installer
Tutorial on Java3D
http://java.sun.com/developer/onlineTraining/java3d/
Procedure for Java3D programming
1. Create a Canvas3D object
2. Create a VirtualUniverse object
3. Create a Locale object, attaching it to the VirtualUniverse object
4. Construct a view branch graph (Major effort is required)
a. Create a View object
b. Create a ViewPlatform object
c. Create a PhysicalBody object
d. Create a PhysicalEnvironment object
e. Attach ViewPlatform, PhysicalBody, PhysicalEnvironment, and Canvas3D objects to Viewobject
5. Construct content branch graph(s)
6. Compile branch graph(s)
7. Insert subgraphs into the Locale
Procedure using SimpleUniverse
1. Create a Canvas3D Object
2. Create a SimpleUniverse object which references the earlier Canvas3D object
a. Customize the SimpleUniverse object
3. Construct content branch
4. Compile content branch graph
5. Insert content branch graph into the Locale of the SimpleUniverse
Sunday, March 4, 2007
Methods of String class
length()
indexOf(int ch)
indexOf(String str, int fromIndex)
lastIndexOf( )
charAt(int index)
substring(int beginindex)
substring(int begin, int end)
toUpperCase()
toLowerCase()
trim()
valueOf( ) 주어진 기본형과 객체를 문자열로 전환
Tuesday, January 16, 2007
Java for Bioinformatics, BioJava
1. 서열정보
* BioJava의 서열 관련 클래스는 모두 index가 1부터 시작된다.
SymbolList 클래스
: 각 서열 Alphabet을 구성하는 Symbol 객체를 가리키는 참조변수로 이루어진다.
따라서, Symbol 객체(DNA, RNA, Protein)가 정의되어 있어야 하고, 아래와 같이 접근.
FiniteAlphabet dna=DNATools.getDNA();
FiniteAlphabet rna=DNATools.getRNA();
FiniteAlphabet protein=ProteinTools.getAlphabet();
Exam)SymbolList 이용한 서열 객체 생성
import org.biojava.bio.seq.*;
import org.biojava.bio.symbol.*;
try{
SymbolList dan=DNATools.createDNA("agtagcgcgta");
}catch(IllegalSymbolException ex){}
Exam)SymbolList 객체를 구성하는 Symbol 객체의 이름 접근
SymbolList dan=DNATools.createDNA("agtagcgcgta");
for(int i=1;i<=dna.length();i++){ //index는 1부터 시작한다. 0이 아니다.
Symbol base=dna.symbolAt(i); // i번째 SymbolList 참조자가 가리키는 Symbol 객체 반환
System.out.println(base.getName()); //Symbol base의 이름 출력(예, a=adenine)
}
Sequence 클래스
:SymbolList와 달리 생물학적 서열을 표현하기 위한 여러 abstract method를 가진다.
Exam) Sequence 객체 생성
try{
Sequence dna=DNAtools.createDNASequence("agtagcgat","DNA_sequence");
}
Exam) FASTA file 읽기
try{
BufferedReader read=new BufferedReader(new FileReader(filename));
SequenceIterator seq=SeqIOTools.readFastaDNA(read); // Genbank 파일일 경우 readGenbank() 이용.
while(seq.hasNext()){
Sequence dna=seq.nextSequence();
//출력 or other 작업
}
}
2. Annotation을 위한 class
Annotation 클래스
:SeqIOTools로 읽은 서열 정보는 각 Feature에 따라 객체에 저장되며,
다양한 Method들을 통해 이들에 접근할 수 있다.
Exam) Genbank 파일의 각 Feature에 따른 정보 출력
SequenceIterator seq=SeqIOTools.readGenbankDNA(read);
while(seq.hasNext()){
Sequence dna=seq.nextSequence();
Annotation anno=dna.getAnnotation();
Iterator key=anno.keys().iterator();
while(key.hasNext()){
Object ob=key.next();
System.out.println(ob.toString()+"="+anno.getProperty(ob));
}
}
Feature 클래스
:하나의 feature에도 위치 정보등 여러 unit의 정보가 저장된다. BioJava에서는 feature 항목과
이에 해당하는 정보 자체를 모두 저장하고, Feature 클래스를 통해 각각 접근할 수 있다.
Exam)위의 exam과 같은 context에서 feature 정보 출력
while(seq.hasNext()){
Sequence dna=seq.nextSequence();
Iterator feature_iter=dna.features();
while(feature_iter.hasNext()){
Feature f=(Feature)(feature_iter.next());
Annotation anno=f.getAnnotation();
System.out.println(f.getType()+"="+anno); //feature type(예, species, sequence, LOCUS)과 이에 해당하는 annotaion 출력
}
}
3. PDB file parsing
import org.biojava.bio.structure.*;
import org.biojava.bio.structure.io.*;
try{
PDBFileReader read=new PDBFileReader();
Structure pdb=read.getStructure(seqfile);
System.out.println("PDB code :"+pdb.getPDBCode());
for(int i=0;i< pdb.nrModels();i++){
ArrayList chains=pdb.getChains(i);
for(Iterator iter=chains.iterator();iter.hasNext();){
Chain c=(Chain)(iter.next());
System.out.println("Chain :"+c.getName()+"\n"+c.getSequence());
Atom a=c.getGroup(0).getAtom(0);
System.out.println("a.getPDBserial()+a.getX()+a.getY()+a.getZ());
}
}
}catch(IOException){}
catch(StructureException){}
Java for Bioinformatics, File parsing
: 공백 문자나 사용자가 지정한 문자를 이용, 문자열을 여러 개의 token으로 쪼개어 준다.
Perl의 split과 같은 기능.
Exam) Genbank 파일을 읽어 Accession number 추출
//Reading file..
String a;
StringTokenizer token=new StringTokenizer(line);
if(token.hasMoreTokens()){
a=token.nextToken();
if(a.equals("ACCESSION")){
System.out.println(token.nextToken());
}
}
Exam2) 구분자 지정
Default 구분자는 공백(\s).
StringTokenizer a=new StringTokenizer(line,"\t"); // tab을 구분자로
StringTokenizer a=new StringTokenizer(line,"\t",true); // tab을 구분자로하고, 구분자까지 token으로 저장
Monday, January 15, 2007
2007 Weight training plan
Body weight : 62.2kg
2007년 1월 현재
Height: 186cm
Body weight : 70kg
Record for three power lifting exercises
2. Deadlift : 100kg(5rm)
3. Bench press: 60kg(3rm)
*Chin up : 10 repeat at one trial, 25 repeat with split trial
2007년 상반기 목표
Body weight : 75kg
Squat : 120kg
Deadlift : 140kg
Bench press: 80kg
Java for Bioinformatics, File I/O
exam) 파일 복사
FileInputStream input;
FileOutputStream output;
String file1=args[0];
String file2=args[1];
try{
input=new FileInputStream(file1);
output=new FileOutputStream(file2);
int b;
while((b=input.read())!=-1){
output.write(b);
}
input.close();
output.close();
}catch(IOException e){
//
e.printStackTrace();
}
버퍼를 이용한 파일 입출력
미리 일정량의 데이터를 읽어 메모리(버퍼)에 저장하는 방법을 사용하여
속도의 향상을 가져온다.
exam) 파일 복사
try{
FileInputStream input=new FileInputStream(args[0]);
FileOutputStream output=new FileOutputStream(args[1]);
BufferedInputStream bufferinput=new BufferedInputStream(input);
BufferedOutputStream bufferoutput=new BufferedOutputStream(output);
int b;
while((b=input.read())!=-1){
output.write(b);
}
input.close();
output.close();
}catch(){}
텍스트 파일 입출력을 위한 Reader/Writer
앞서의 Fileinoutstream or fileoutputstream이 byte단위로 데이터를 처리했던데
반해, 16 bit UNICODE 문자 단위로 처리한다. 한글의 경우 16bit로 표현되므로,
앞서의 경우처럼 byte(8bit)단위로 읽었을 때는 반쪽만 읽어 반환하여 문자가
깨지는 경우가 발생한다.
exam)
try{
FileReader read=new FileReader(args[0]);
int b;
while((b=read.read())!=-1){
System.out.println((char)b);
}
read.close();
}
exam2) 버퍼 이용
try{
FileReader read=new FileReader(args[0]);
BufferedReader r=new BufferedReader(read);
String line;
while((line=r.readLine())!=null){
System.out.println(line);
}
read.close();
r.close();
}
newLine() 메소드 // BufferedWrite 객체에 '\n'을 넣어준다.
Byte 스트림을 문자 스트림으로 변환
InputStreamReader를 통해 바이트 스트림이 문자 스트림으로,
OutputStreamWriter을 통해 문자 스트림이 바이트 스트림으로 변환된다.
try{
FileInputStream input=new FileInputStream(args[0]);
FileOutputStream output=new FileOutputStream(args[1]);
InputStreamReader reader=new InputStreamReader(input);
OutputStreamWriter writer=new OutputStreamWriter(output);
int b;
while((b=reader.read())!=-1){
writer.write(b);
}
}
FASTA file 읽기 예제
String name;
String seq;
StringBuffer seqbuf=new StringBuffer();
try{
BufferedReader read=new BufferedReader(new FileReader(args[0]));
while((line=read.readLine())!=null){
line=line.trim();
if(line.length()==0) continue;
if(line.charAt(0)=='>'){
name=line.substring(1); // >enzyme1 에서 위치 1 이후의 문자열 enzyme1을 반환
}else{
seqbuf.append(line);
}
read.close();
seq=seqbuf.toString();
}
}
인터넷 resourse 읽어들이기
java.net 패키지의 URLConnection 클래스 이용.
exam)
import java.net.*;
String urlstr="http://~~"
URL url=new URL(urlstr);
URLConnection c=url.openConnection();
BufferedReader re=new BufferedReader(new InputStreamReader(c.getInputStream());
Java for Bioinformatics, chap. 2 through 5
break를 통해 break 될 블록을 지정할 수 있다.
Exam)
test: for( .. ){
for( ..) {
if( ..)
break test;
}
}
String 클래스 메소드들
1. CharAt()
String 클래스의 메소드로 문자열의 특정 위치 문자 반환.
Exam)
String DNA="ATGATGA";
char A=DNA.charAt(1);
A에는 DNA 문자열의 2번째 character인 T가 저장.
2. 문자열 상수
문자열 상수는 String 클래스의 객체로 구현.
따라서
int a="atgatcta".length();
도 가능하다.
3. toUpperCase()
dna.toUpperCase() // 대문자로 변환
4. substring(int beginIndex, int endIndex)
beginIndex부터 endIndex의 앞에서 끝나는 부분의 문자열 반환
or substring(int beginIndex)로 특정 부분부터 끝까지의 문자열 반환
5. 문자열 결합
dna=dna+seq;
식의 + 연산자 이용한 결합 가능
StringBuffer 클래스
String 객체는 문자를 추가하거나 지워서 길이를 변화시킬 수 없고,
이런 기능이 필요할 때 StringBuffer 객체를 쓴다.
Exam)
StringBuffer dna=new StringBuffer();
dna.append('T');
dna.append('A');
dna.reverse(); // 스트링 순서를 뒤집는다.
dna.insert(0,'A'); 0번째 위치에 A를 집어 넣는다.
dna.toString(); // String 객체로 type 변환. (type casting)
입력 (명령어 라인, 실행 중 입력)
String dna=args[0]; //명령어 라인 입력
String dna;
byte[] b=new byte[10000];
try{
System.in.read(b);
dna=new String(b);
dna=dna.trim();
}
catch(Exception e){
//
}
패키지 사용
파일의 상위에 package pakagename;
을 명시하여 같은 package파일 임을 표시해 준다.
자바에서 패키지 이름은 폴더 이름과 대응하고
-d 옵션을 통해 .class 파일들이 생성될 폴더 위치를
정해주면 패키지 이름과 같은 폴더가 만들어진다.
javac -d . *.java
java packagename.mainclassname //실행 클래스의 폴더 위치를 . 앞에 써준다. .는 \를 의미.
생성된 package는 다른 프로그램에서 import 문으로 불러 사용할 수 있다.
Hashtable
Hashtable h=new Hashtable();
h.put('AAA','K');
String codon=(String)h.get('AAA');
Hashtable에 저장되거나 키값으로 사용되는 것은 모두 객체.
따라서 기본 자료형을 사용할 때는 wrapper를 사용해야 한다.
h.put(new Integer(123),new Integer(355));
h.put(new Character('a'),new Character('b');
instanceOf 연산자
boolean=dna instanceOf DNA; //dna 객체가 DNA class의 객체인지를 확인하여 ture or false값을 반환.